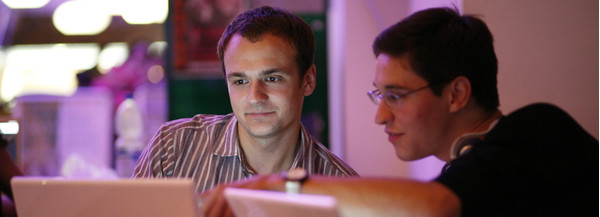
Exploring Continual Learning of Compositional Generalization in NLI
Abstract
Compositional Natural Language Inference has previously been explored to assess the true capabilities of PLMs to perform NLI. However, existing models and evaluations assume full access to all trained data in advance, in contrast to the reality of language learning where humans continually acquire knowledge with more limited access to data samples at a time. In this talk, we propose a novel and challenging task we call Continual Compositional Generalization in Inference (C^2Gen), where a model continually adapts to primitives or noise. With this task, we aim to explore whether and to what extent continual learning will affect compositional generalization. To investigate this question, we decompose compositional inference into two fine-grained constituting sub-tasks: recognition of primitives and compositional reasoning. We benchmark a selection of continual learning algorithms, and analyze i.) a model’s preservation of knowledge about primitives, ii.) its ability to perform compositional generalization, and iii.) the risk of forgetting – under controlled datastreams. Our experimental results highlight a number of effects caused by different orders in the presentation of primitives and noise, implicating a recipe for future compositional inference learning setups and evaluation schemes.