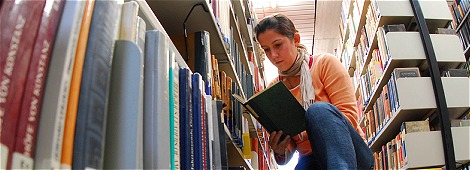
Empirical Methods for NLP and Data Science
Module Description
Course | Module Abbreviation | Credit Points |
---|---|---|
BA-2010 | AS-CL | 8 LP |
Master | SS-CL, SS-TAC | 8 LP |
Seminar Informatik | BA + MA | 4 LP |
Anwendungsgebiet Informatik | MA | 8 LP |
Anwendungsgebiet SciComp | MA | 8 LP |
Lecturer | Stefan Riezler |
Module Type | |
Language | English |
First Session | 26.04.2022 |
Time and Place |
Dienstag, 13:15–14:45 INF 328 / SR25 |
Commitment Period | tbd. |
Prerequisite for Participation
Good knowledge of statistical machine learning (e.g., by successful completion of courses "Statistical Methods for Computational Linguistics" and/or "Neural Networks: Architectures and Applications for NLP") and experience in experimental work (e.g., software project or seminar implementation project)
Assessment
Content
Empirical methods are means to answering methodological questions of empirical sciences by statistical techniques. The methodological questions addressed in this seminar include the problems of validity, reliability, and significance. In the case of machine learning, these correspond to the questions of whether a model predicts what it purports to predict, whether a model's performance is consistent across replications, and whether a performance difference between two models is due to chance, respectively. The goal of this seminar is to answer these questions by concrete statistical tests that can be applied to assess validity, reliability, and significance of data annotation and machine learning prediction in the fields of NLP and data science.
The focus of the class is on model-based empirical methods where data annotations and model predictions are treated as training data for interpretable probabilistic models from the well-understood families of generalized additive models (GAMs) and linear mixed effects models (LMEMs). Based on the interpretable parameters of the trained GAMs or LMEMs, we will investigate model-based statistical tests such as a validity test that allows detecting circular features that circumvent learning. Furthermore, we will discuss reliability coefficients using variance decomposition based on random effect parameters of LMEMs. Last, we investigate significance tests based on the likelihood ratios of nested LMEMs trained on the performance scores of two machine learning models. This test will be shown to naturally allow the inclusion of variations in meta-parameter settings into hypothesis testing, and further facilitates a refined system comparison conditional on properties of input data.
This course serves an introduction to empirical methods for machine learning in general, with a special focus on applications in NLP and data science. It is based on the textbook Validity, Reliability, and Significance: Model-Based Empirical Methods for NLP, by Stefan Riezler and Michael Hagmann, available at:
http://www.morganclaypoolpublishers.com/catalog_Orig/product_info.php?products_id=1688
The book is self-contained with an appendix on the mathematical background on GAMs and LMEMs, and with an accompanying webpage including R code to replicate experiments presented in the book.
A list of further literature will be given in the first session of the seminar.
Literature
Enrollment
Please enroll at the CL enrollment page until April 20, 2022, 23:59.