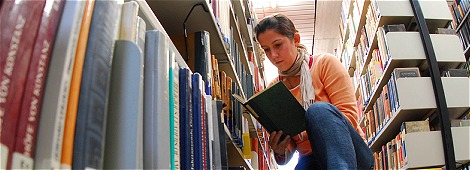
Recent Advances In Sequence-To-Sequence Learning
Module Description
Course | Module Abbreviation | Credit Points |
---|---|---|
BA-2010[100%|75%] | CS-CL | 6 LP |
BA-2010[50%] | BS-CL | 6 LP |
BA-2010[25%] | BS-AC | 4 LP |
BA-2010 | AS-CL | 8 LP |
Master | SS-CL, SS-TAC | 8 LP |
Lecturer | Tsz Kin Lam |
Module Type | |
Language | English |
First Session | 24.10.2019 |
Time and Place | Thursday, 14:15-15:45 INF 326 / SR 27 |
End of Commitment Period | 21.01.2020 |
Prerequisite for Participation
•Basic knowledge in probability, statistics, and linear algebra, e.g. Mathematical Foundations and Statistical Methods for Computational Linguistics. •Basic knowledge in neural networks, e.g., Neural Networks: Architectures and Applications for NLP
Assessment
•Regular and active attendance of seminar •Reading of rest of papers from seminar reading list •Presentation of paper(s) from seminar reading list •Implementation project
Content
Neural Sequence-To-Sequence Learning (Seq2Seq) is about finding mappings between input and the output sequences using neural networks. The “sequence” can be of many different forms, e.g., financial time series, DNA, audio signals, texts, and images. The two sequences can be of the same or different modality, leading to various applications such as textual or spoken machine translation, text summarisation, caption generation, and semantic parsing.
Seq2Seq is a fully data-driven approach. It has been empirically shown to perform better than traditional statistical learning approaches in many scenarios, especially on large-scale problems. Additionally, it is end-to-end which naturally prevents error propagation as happening in cascaded systems.
In this seminar, we will discuss recent advances in Seq2Seq learning with three main themes centred around Machine Translation. We first start from (1) Neural Network Architectures for Seq2Seq, e.g., ConvSeq2Seq, Transformer and RNMT+. We then move to (2) Low-resources setting with strong focus on leveraging unpaired data, e.g., Fusion Techniques, Back-Translation and Dual Learning. Finally, (3) we will break the left-to-right generation order and move to a novel regime, called Non-autoregressive Neural Sequence Generation, e.g., Levenshtein Transformer and Insertion Transformer.